视频 API#
本示例展示了 torchvision 为视频提供的一些 API,以及如何构建数据集等的示例。
介绍:构建新的视频对象并检查其属性#
首先选择视频来测试对象。为了便于讨论,使用 kinetics400 数据集中的视频。要创建它,需要定义路径和要使用的流。
选择的视频统计信息:
WUzgd7C1pWA.mp4
source:
kinetics-400
video:
H-264
MPEG-4 AVC (part 10) (avc1)
fps: 29.97
audio:
MPEG AAC audio (mp4a)
sample rate: 48K Hz
from set_env import temp_dir
项目根目录:/media/pc/data/lxw/ai/torch-book
import torch
import torchvision
from torchvision.datasets.utils import download_url
torchvision.set_video_backend("video_reader")
# Download the sample video
download_url(
"https://raw.githubusercontent.com/pytorch/vision/refs/heads/main/test/assets/videos/WUzgd7C1pWA.mp4",
temp_dir,
"WUzgd7C1pWA.mp4"
)
video_path = f"{temp_dir}/WUzgd7C1pWA.mp4"
Downloading https://raw.githubusercontent.com/pytorch/vision/refs/heads/main/test/assets/videos/WUzgd7C1pWA.mp4 to /media/pc/data/lxw/ai/torch-book/tests/.temp/tasks/WUzgd7C1pWA.mp4
100%|██████████| 890k/890k [00:00<00:00, 1.05MB/s]
流以类似于 torch 设备的方式定义。我们将它们编码为 stream_type:stream_id
形式的字符串,其中 stream_type
是字符串,stream_id
是长整数。构造函数接受仅传递 stream_type
,在这种情况下,流会自动发现。首先,让我们获取特定视频的元数据:
stream = "video"
video = torchvision.io.VideoReader(video_path, stream)
video.get_metadata()
{'video': {'duration': [10.9109], 'fps': [29.97002997002997]},
'audio': {'duration': [10.9], 'framerate': [48000.0]},
'subtitles': {'duration': []},
'cc': {'duration': []}}
在这里可以看到视频有两个流 - 一个视频流和一个音频流。目前可用的流类型包括 ['video', 'audio']
。每个描述符由两部分组成:流类型(例如 'video'
)和唯一的流 ID(由视频编码确定)。通过这种方式,如果视频容器包含多个相同类型的流,用户可以访问他们想要的流。如果仅传递流类型,解码器会自动检测该类型的第一个流并返回它。
读取视频流中的所有帧。默认情况下,next(video_reader)
的返回值是一个包含以下字段的字典。
返回字段是:
data
:包含一个 torch.tensorpts
:包含此特定帧的浮点时间戳
metadata = video.get_metadata()
video.set_current_stream("audio")
frames = [] # we are going to save the frames here.
ptss = [] # pts is a presentation timestamp in seconds (float) of each frame
for frame in video:
frames.append(frame['data'])
ptss.append(frame['pts'])
print("PTS for first five frames ", ptss[:5])
print("Total number of frames: ", len(frames))
approx_nf = metadata['audio']['duration'][0] * metadata['audio']['framerate'][0]
print("Approx total number of datapoints we can expect: ", approx_nf)
print("Read data size: ", frames[0].size(0) * len(frames))
PTS for first five frames [0.0, 0.021332999999999998, 0.042667, 0.064, 0.08533299999999999]
Total number of frames: 511
Approx total number of datapoints we can expect: 523200.0
Read data size: 523264
但如果只想读取视频的特定时间段呢?这可以通过结合我们的 seek
函数和每次调用 next 返回的帧的呈现时间戳(以秒为单位)来轻松完成。
鉴于实现依赖于 Python 迭代器,可以利用 itertools 来简化过程并使其更具 Python 风格。
例如,如果想从第二秒开始读取十帧:
import itertools
video.set_current_stream("video")
frames = [] # we are going to save the frames here.
# We seek into a second second of the video and use islice to get 10 frames since
for frame, pts in itertools.islice(video.seek(2), 10):
frames.append(frame)
print("Total number of frames: ", len(frames))
Total number of frames: 10
或者如果想从第二秒到第五秒读取,我们定位到视频的第二秒,然后我们利用 itertools takewhile 来获取正确数量的帧:
video.set_current_stream("video")
frames = [] # we are going to save the frames here.
video = video.seek(2)
for frame in itertools.takewhile(lambda x: x['pts'] <= 5, video):
frames.append(frame['data'])
print("Total number of frames: ", len(frames))
approx_nf = (5 - 2) * video.get_metadata()['video']['fps'][0]
print("We can expect approx: ", approx_nf)
print("Tensor size: ", frames[0].size())
Total number of frames: 90
We can expect approx: 89.91008991008991
Tensor size: torch.Size([3, 256, 340])
构建示例 read_video 函数#
可以利用上述方法来构建遵循与现有 read_video
函数相同 API 的 read_video 函数。
def example_read_video(video_object, start=0, end=None, read_video=True, read_audio=True):
if end is None:
end = float("inf")
if end < start:
raise ValueError(
"end time should be larger than start time, got "
f"start time={start} and end time={end}"
)
video_frames = torch.empty(0)
video_pts = []
if read_video:
video_object.set_current_stream("video")
frames = []
for frame in itertools.takewhile(lambda x: x['pts'] <= end, video_object.seek(start)):
frames.append(frame['data'])
video_pts.append(frame['pts'])
if len(frames) > 0:
video_frames = torch.stack(frames, 0)
audio_frames = torch.empty(0)
audio_pts = []
if read_audio:
video_object.set_current_stream("audio")
frames = []
for frame in itertools.takewhile(lambda x: x['pts'] <= end, video_object.seek(start)):
frames.append(frame['data'])
audio_pts.append(frame['pts'])
if len(frames) > 0:
audio_frames = torch.cat(frames, 0)
return video_frames, audio_frames, (video_pts, audio_pts), video_object.get_metadata()
# Total number of frames should be 327 for video and 523264 datapoints for audio
vf, af, info, meta = example_read_video(video)
print(vf.size(), af.size())
torch.Size([327, 3, 256, 340]) torch.Size([523264, 1])
3. 构建示例随机采样数据集(可应用于 kinetics400 的训练数据集)#
酷,现在可以使用相同的原则来制作样本数据集。我们建议为此目的尝试可迭代数据集。在这里,将构建示例数据集,读取随机选择的 10 帧视频。
制作样本数据集:
(temp_dir/"./dataset").mkdir(exist_ok=True)
(temp_dir/"./dataset/1").mkdir(exist_ok=True)
(temp_dir/"./dataset/2").mkdir(exist_ok=True)
下载视频:
from torchvision.datasets.utils import download_url
root_url = "https://raw.githubusercontent.com/pytorch/vision/refs/heads/main/test/assets/videos"
download_url(
f"{root_url}/WUzgd7C1pWA.mp4?raw=true",
temp_dir/"./dataset/1", "WUzgd7C1pWA.mp4"
)
download_url(
f"{root_url}/RATRACE_wave_f_nm_np1_fr_goo_37.avi?raw=true",
temp_dir/"./dataset/1",
"RATRACE_wave_f_nm_np1_fr_goo_37.avi"
)
download_url(
f"{root_url}/SOX5yA1l24A.mp4?raw=true",
temp_dir/"./dataset/2",
"SOX5yA1l24A.mp4"
)
download_url(
f"{root_url}/v_SoccerJuggling_g23_c01.avi?raw=true",
temp_dir/"./dataset/2",
"v_SoccerJuggling_g23_c01.avi"
)
download_url(
f"{root_url}/v_SoccerJuggling_g24_c01.avi?raw=true",
temp_dir/"./dataset/2",
"v_SoccerJuggling_g24_c01.avi"
)
Downloading https://raw.githubusercontent.com/pytorch/vision/refs/heads/main/test/assets/videos/WUzgd7C1pWA.mp4?raw=true to /media/pc/data/lxw/ai/torch-book/tests/.temp/tasks/dataset/1/WUzgd7C1pWA.mp4
100%|██████████| 890k/890k [00:00<00:00, 1.17MB/s]
Downloading https://raw.githubusercontent.com/pytorch/vision/refs/heads/main/test/assets/videos/RATRACE_wave_f_nm_np1_fr_goo_37.avi?raw=true to /media/pc/data/lxw/ai/torch-book/tests/.temp/tasks/dataset/1/RATRACE_wave_f_nm_np1_fr_goo_37.avi
100%|██████████| 264k/264k [00:00<00:00, 608kB/s]
Downloading https://raw.githubusercontent.com/pytorch/vision/refs/heads/main/test/assets/videos/SOX5yA1l24A.mp4?raw=true to /media/pc/data/lxw/ai/torch-book/tests/.temp/tasks/dataset/2/SOX5yA1l24A.mp4
100%|██████████| 560k/560k [00:00<00:00, 1.41MB/s]
Downloading https://raw.githubusercontent.com/pytorch/vision/refs/heads/main/test/assets/videos/v_SoccerJuggling_g23_c01.avi?raw=true to /media/pc/data/lxw/ai/torch-book/tests/.temp/tasks/dataset/2/v_SoccerJuggling_g23_c01.avi
100%|██████████| 508k/508k [00:00<00:00, 1.44MB/s]
Downloading https://raw.githubusercontent.com/pytorch/vision/refs/heads/main/test/assets/videos/v_SoccerJuggling_g24_c01.avi?raw=true to /media/pc/data/lxw/ai/torch-book/tests/.temp/tasks/dataset/2/v_SoccerJuggling_g24_c01.avi
100%|██████████| 623k/623k [00:00<00:00, 711kB/s]
家政服务与公共事业:
import os
import random
from torchvision.datasets.folder import make_dataset
from torchvision import transforms as t
def _find_classes(dir):
classes = [d.name for d in os.scandir(dir) if d.is_dir()]
classes.sort()
class_to_idx = {cls_name: i for i, cls_name in enumerate(classes)}
return classes, class_to_idx
def get_samples(root, extensions=(".mp4", ".avi")):
_, class_to_idx = _find_classes(root)
return make_dataset(root, class_to_idx, extensions=extensions)
定义数据集和一些基本参数。假设 FolderDataset 的结构,并添加以下参数:
clip_len
:剪辑的帧长度frame_transform
:每个帧的变换video_transform
:视频序列的变换
备注
实际上添加了 epoch 大小,因为使用 IterableDataset()
类允许我们根据需要从每个视频中自然地过度采样剪辑或图像。
class RandomDataset(torch.utils.data.IterableDataset):
def __init__(self, root, epoch_size=None, frame_transform=None, video_transform=None, clip_len=16):
super(RandomDataset).__init__()
self.samples = get_samples(root)
# Allow for temporal jittering
if epoch_size is None:
epoch_size = len(self.samples)
self.epoch_size = epoch_size
self.clip_len = clip_len
self.frame_transform = frame_transform
self.video_transform = video_transform
def __iter__(self):
for i in range(self.epoch_size):
# Get random sample
path, target = random.choice(self.samples)
# Get video object
vid = torchvision.io.VideoReader(path, "video")
metadata = vid.get_metadata()
video_frames = [] # video frame buffer
# Seek and return frames
max_seek = metadata["video"]['duration'][0] - (self.clip_len / metadata["video"]['fps'][0])
start = random.uniform(0., max_seek)
for frame in itertools.islice(vid.seek(start), self.clip_len):
video_frames.append(self.frame_transform(frame['data']))
current_pts = frame['pts']
# Stack it into a tensor
video = torch.stack(video_frames, 0)
if self.video_transform:
video = self.video_transform(video)
output = {
'path': path,
'video': video,
'target': target,
'start': start,
'end': current_pts}
yield output
给定文件夹结构中的视频路径,即:
dataset
class 1
file 0
file 1
…
class 2
file 0
file 1
…
…
可以生成数据加载器并测试数据集。
transforms = [t.Resize((112, 112))]
frame_transform = t.Compose(transforms)
dataset = RandomDataset(f"{temp_dir}/dataset", epoch_size=None, frame_transform=frame_transform)
from torch.utils.data import DataLoader
loader = DataLoader(dataset, batch_size=12)
data = {"video": [], 'start': [], 'end': [], 'tensorsize': []}
for batch in loader:
for i in range(len(batch['path'])):
data['video'].append(batch['path'][i])
data['start'].append(batch['start'][i].item())
data['end'].append(batch['end'][i].item())
data['tensorsize'].append(batch['video'][i].size())
print(data)
{'video': ['/media/pc/data/lxw/ai/torch-book/tests/.temp/tasks/dataset/2/SOX5yA1l24A.mp4', '/media/pc/data/lxw/ai/torch-book/tests/.temp/tasks/dataset/2/v_SoccerJuggling_g24_c01.avi', '/media/pc/data/lxw/ai/torch-book/tests/.temp/tasks/dataset/1/RATRACE_wave_f_nm_np1_fr_goo_37.avi', '/media/pc/data/lxw/ai/torch-book/tests/.temp/tasks/dataset/2/v_SoccerJuggling_g23_c01.avi', '/media/pc/data/lxw/ai/torch-book/tests/.temp/tasks/dataset/1/RATRACE_wave_f_nm_np1_fr_goo_37.avi'], 'start': [5.627342589083136, 5.1310869183540735, 0.11882101882870964, 4.3466401676540025, 0.8623983005356067], 'end': [6.139467, 5.638967, 0.6333329999999999, 4.8715329999999994, 1.3666669999999999], 'tensorsize': [torch.Size([16, 3, 112, 112]), torch.Size([16, 3, 112, 112]), torch.Size([16, 3, 112, 112]), torch.Size([16, 3, 112, 112]), torch.Size([16, 3, 112, 112])]}
数据可视化#
可视化视频的示例
import matplotlib.pyplot as plt
plt.figure(figsize=(12, 12))
for i in range(16):
plt.subplot(4, 4, i + 1)
plt.imshow(batch["video"][0, i, ...].permute(1, 2, 0))
plt.axis("off")
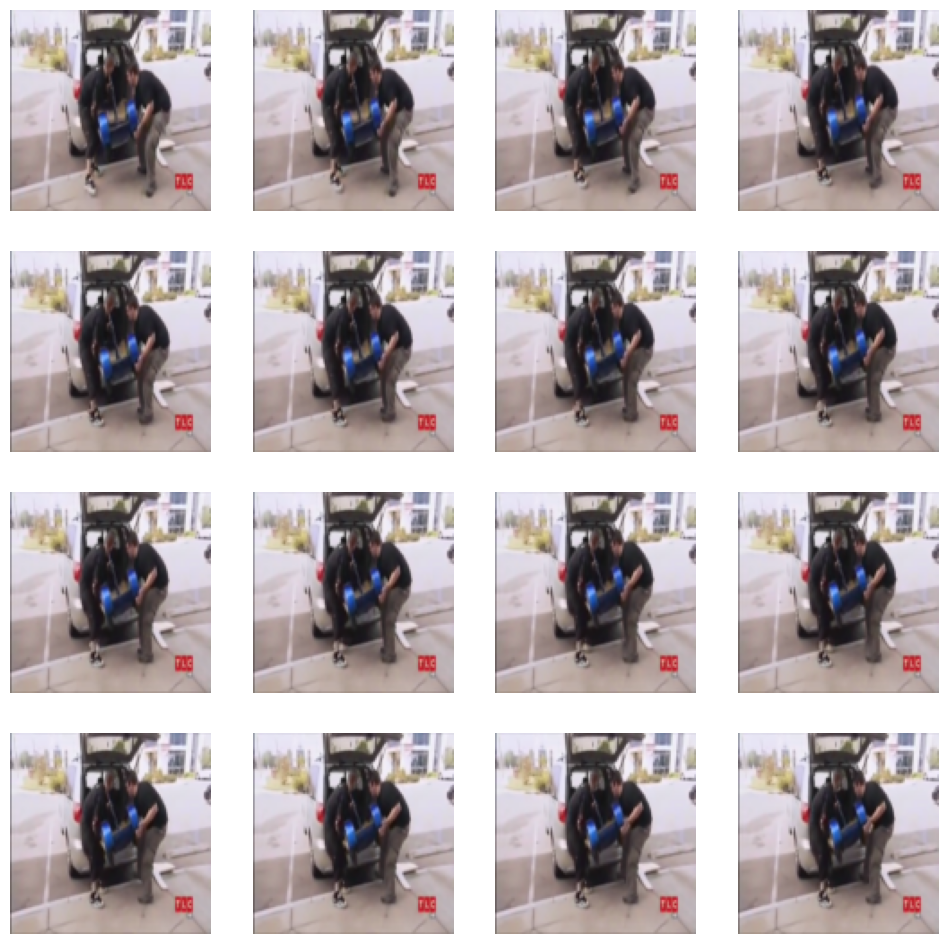
清理视频和数据集:
import os
import shutil
os.remove(temp_dir/"./WUzgd7C1pWA.mp4")
shutil.rmtree(temp_dir/"./dataset")