快速上手 torchvision.transforms.v2
#
首先,进行一些设置
from pathlib import Path
import torch
from matplotlib import pyplot as plt
plt.rcParams["savefig.bbox"] = 'tight'
from torchvision.transforms import v2
from torchvision.io import read_image
torch.manual_seed(1)
# If you're trying to run that on collab, you can download the assets and the
# helpers from https://github.com/pytorch/vision/tree/main/gallery/
from utils.helpers import plot
img = read_image(str(Path('../assets') / 'astronaut.jpg'))
print(f"{type(img) = }, {img.dtype = }, {img.shape = }")
type(img) = <class 'torch.Tensor'>, img.dtype = torch.uint8, img.shape = torch.Size([3, 512, 512])
v2 基础#
Torchvision 转换的行为就像常规的 torch.nn.Module
(事实上,它们中的大多数都是):实例化转换,传递输入,得到转换后的输出:
transform = v2.RandomCrop(size=(224, 224))
out = transform(img)
plot([img, out])
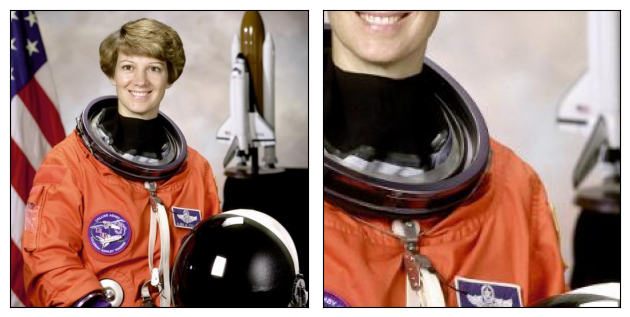
简单的分类管道#
transforms = v2.Compose([
v2.RandomResizedCrop(size=(224, 224), antialias=True),
v2.RandomHorizontalFlip(p=0.5),
v2.ToDtype(torch.float32, scale=True),
v2.Normalize(mean=[0.485, 0.456, 0.406], std=[0.229, 0.224, 0.225]),
])
out = transforms(img)
plot([img, out])
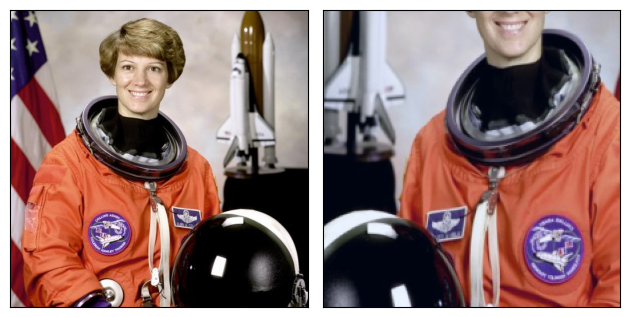
这样的转换管道通常作为 transform
参数传递给 Datasets <datasets>
,例如 ImageNet(..., transform=transforms)
。
检测、分割、视频#
torchvision.transforms.v2
命名空间中的新的 Torchvision 转换支持图像分类以外的任务:它们还可以转换边界框、分割/检测掩码或视频。
让我们简要地看一下带有边界框的检测示例。
from torchvision import tv_tensors # we'll describe this a bit later, bare with us
boxes = tv_tensors.BoundingBoxes(
[
[15, 10, 370, 510],
[275, 340, 510, 510],
[130, 345, 210, 425]
],
format="XYXY", canvas_size=img.shape[-2:])
transforms = v2.Compose([
v2.RandomResizedCrop(size=(224, 224), antialias=True),
v2.RandomPhotometricDistort(p=1),
v2.RandomHorizontalFlip(p=1),
])
out_img, out_boxes = transforms(img, boxes)
print(type(boxes), type(out_boxes))
plot([(img, boxes), (out_img, out_boxes)])
<class 'torchvision.tv_tensors._bounding_boxes.BoundingBoxes'> <class 'torchvision.tv_tensors._bounding_boxes.BoundingBoxes'>
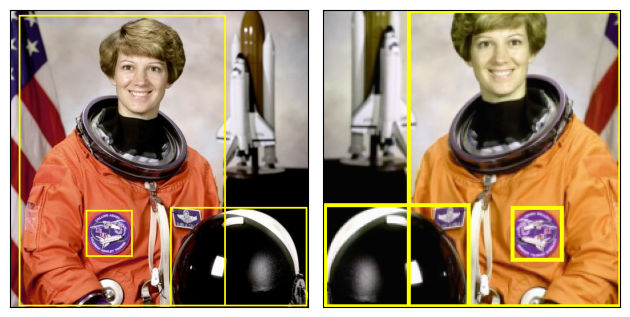
上述示例主要关注目标检测。但是,如果我们有用于对象分割或语义分割的掩码(torchvision.tv_tensors.Mask
)或视频(torchvision.tv_tensors.Video
),我们可以以完全相同的方式将它们传递给转换。
现在您可能有几个问题:这些 TVTensors 是什么,我们如何使用它们,以及这些转换的预期输入/输出是什么?我们将在下一节中回答这些问题。
TVTensors 是什么?#
TVTensors 是 torch.Tensor
子类,可得到 Image
、BoundingBoxes
、Mask
和 Video
。
TVTensors 看起来和感觉就像普通的张量一样 - 它们就是张量。像 .sum()
或任何 torch.*
算子这样的普通 torch.Tensor
支持的所有内容也将在 TVTensor 上工作:
img_dp = tv_tensors.Image(torch.randint(0, 256, (3, 256, 256), dtype=torch.uint8))
print(f"{isinstance(img_dp, torch.Tensor) = }")
print(f"{img_dp.dtype = }, {img_dp.shape = }, {img_dp.sum() = }")
isinstance(img_dp, torch.Tensor) = True
img_dp.dtype = torch.uint8, img_dp.shape = torch.Size([3, 256, 256]), img_dp.sum() = tensor(25087958)
这些 TVTensor 类是转换的核心:为了转换给定的输入,转换首先查看对象的类,并相应地分派到适当的实现。
在这个阶段,您不需要了解更多关于 TVTensors 的知识,但希望了解更多的高级用户可以参考 TVTensors FAQ。
需要传递什么作为输入?#
在上面,我们看到了两个示例:一个将单个图像作为输入,即 out = transforms(img)
,另一个同时传递了图像和边界框,即 out_img, out_boxes = transforms(img, boxes)
。
实际上,转换支持任意输入结构。输入可以是单个图像、元组、任意嵌套的字典…几乎任何东西。相同的结构将作为输出返回。下面,我们使用相同的检测转换,但将元组(图像,目标字典)作为输入,并获得与输出相同的结构:
target = {
"boxes": boxes,
"labels": torch.arange(boxes.shape[0]),
"this_is_ignored": ("arbitrary", {"structure": "!"})
}
# Re-using the transforms and definitions from above.
out_img, out_target = transforms(img, target)
plot([(img, target["boxes"]), (out_img, out_target["boxes"])])
print(f"{out_target['this_is_ignored']}")
('arbitrary', {'structure': '!'})
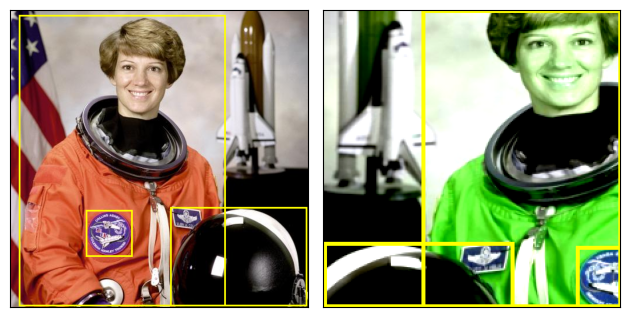
我们传递了一个元组,所以我们得到了一个元组作为输出,第二个元素是转换后的目标字典。转换并不真正关心输入的结构;如上所述,它们只关心对象的类型并相应地进行转换。
对于像字符串或整数这样的外部对象,它们会原样传递。这在调试时可能会很有用,例如,如果你想为每个样本关联一个路径!
转换和数据集的兼容性#
粗略地说,数据集的输出必须与转换的输入相对应。如何做到这一点取决于您是使用 torchvision 内置的数据集还是自己的自定义数据集。
内置的数据集#
如果您只是进行图像分类,则无需执行任何操作。只需使用数据集的 transform
参数,例如 ImageNet(..., transform=transforms)
,然后就可以开始了。
Torchvision 还支持像 torchvision.datasets.CocoDetection
这样的目标检测或分割数据集。这些数据集比 torchvision.transforms.v2
模块和 TVTensors 更早出现,因此它们不会自动返回 TVTensors。强制使这些数据集返回 TVTensors 并使它们与 v2 转换兼容的简单方法是使用 torchvision.datasets.wrap_dataset_for_transforms_v2()
函数:
from torchvision.datasets import CocoDetection, wrap_dataset_for_transforms_v2
dataset = CocoDetection(..., transforms=my_transforms)
dataset = wrap_dataset_for_transforms_v2(dataset)
# Now the dataset returns TVTensors!